Every decade, a new Billion-dollar data analytics company is born. In the early 2000s, Tableau was one. In the previous decade, it was Looker. What and who is going to be such a company this decade?
It should be obvious, to anyone who is looking, that introduction of Generative AI to the data landscape is going to have significant implications. One of those opportunities is the idea of AI Data Developer: AI that doesn't just generate a codebase, but produces that initial draft and then offers an interactive environment where users—maybe analysts and data developers—can give it feedback (see here).
When I previously shared at various events that this is indeed what we’re working on, someone would inevitably jump out—as if ordained by God—and would exclaim: “but, wait, what’s the Moat?” And then, as if their inner-toddler just woke up, their gaze would start to wonder around the room, looking for the next victim - typically someone with just enough idealism; someone eager to persuade the non-believers.
A Thought Experiment: I am the Idealist - and You, well, the Non-believer. Let’s go!
When one is pitching investors, it is important to paint a picture of the infinite potential. For example, when we explained our product to our current investors, we highlighted that the tool helps address the cost of talent in the data space - something that is becoming prohibitive as more and more companies draw on a relatively small pool of specialists.
But what such basic analysis of AI-for-Data start-ups often misses is that for the majority of companies trying to get value of data, the potential derives from basic unit economics. I previously wrote about this in my comparison of restaurants and data software companies. The gist: you can slice and dice data a thousand different ways, but in the end, just like the meat in the burger, data has a basic unit of value which you cannot exceed.
One such example is that of a credit card processing company (think Visa or MasterCard). If such a company makes 30c on every transaction - short of some magic, the value of the data analytics tool will always have an upper limit of <30c per record in the database. It is like a physical property of an atom. All of us can dream, but we have to respect the laws of physics.
For all the shine and glow, data vendors are in the commodity business. And don’t let any sleazy salesman tell you anything different. The value of the AI in the Data Analytics stack too does not just magically make 30c a dollar.
Yet, there can still be a MOAT. In fact, MOAT in a commodity business is what it is all about. Stick with me here…
Commodity Ideas have the largest MOAT
Do you know who else is in the commodity business? That’s right, BiC - the company that even in the age of computers manages to sell pencils ✏️ and bring north of $2billion in revenue per year.
Commodity does not mean lack of differentiation. The video at the top of this newsletter demonstrates the process a Japanese manufacturer uses to produce pencils (if your browser does not show it - enable it - it is worth it, I promise!). The process is known. You and I can start such a business. And yet, it is not likely that either of us would make money. But BiC does! I could try to point to some trick in BiC’s business model, but let’s face it - there is not one magical trick. They know distribution; they know manufacturing; they have a diverse line of cheap but reliable products; and they know this business. It seems so simple, and yet it is really not.
The point here is that it is not enough just to know at a high level how a system is build. Elon Musk’s Tesla famously open-sourced the designs for Tesla Roadster 🏎. But many years later, Tesla still remains a much more superior Electric vehicle. Some have the hardware, but lack the software. Others have the software and the hardware, but lack the network of charging stations. And nobody except Tesla has all of it.
Applications Guarantee Quality - Generic AI Providers Do NOT
An investor once asked me what size businesses are we going after. In my response I stated that we’re initially going after businesses with at least 1,000 employees because that’s where they likely have enough scale and maturity to feel the most pain. To this the investor’s associate replied that there are very few companies of such size 🙄. “Incompetent Analyst!” - screamed my inner voice. (Rule #1: don’t bring bad data arguments to a Founder of a DATA Company).
ChatGPT to the rescue, I wrongly thought and asked. ChatGPT came back with some nonsensical answer. Not to look weak, I asked it again - only to receive the same verbose nonsensical answer. The opportunity for the effect disappeared, but, of course, my argument was sound: a quick LinkedIn employer search returns 12,920 such companies in the USA (10,275+1,437+795+413) for combined categories of >1,000 employees and only 11,991 companies for employers with 500 to 1000 employees (there are lots more small businesses with <500 employees). The associate wanted us to disregard 50% of the market with 80-95% of the money 👏.
But in the midst of this exercise, a much stronger argument emerged for this new breed of AI Application companies. An AI application designed around market research would never had failed as miserably as ChatGPT did during my conversation with the said investor. An application based on RAG and some recommender system, would have tailored the scope to one based on simple pre-defined sources - like one I used myself manually - and would have retrieved an answer from a much more defined set of end result options, creating the answer that is both specific and accurate.
The simple truth is: raw cloud providers have a contract around usage and feature availability, but not the quality of the end-user experience.
raw cloud providers have a contract around usage and feature availability, but not the quality of the end-user experience.
Who else has a contract around the quality of end-user experience? BiC and Tesla do.
Delivering a Great Product!
So how do you deliver a great product? One that has a moat. One that is like one of those BiC pens or pencils, delivers tons of value for a fraction of the cost.
Watch this video. The answer is so simple. Well, maybe.
Happy Friday!
About the Author
In my former life I was a Data Scientist. I studied Computer Science and Economics for undergrad and then Statistics in graduate school, eventually joining a small venture-backed Series A company in Santa Cruz, CA. That company’s name was Looker - a Google Cloud company.
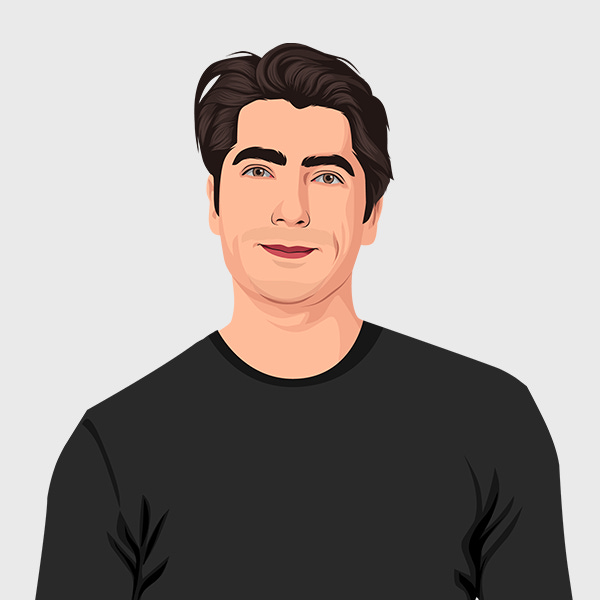
Mir's Data .Report is a reader-supported publication.
What's the MOAT?